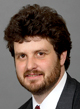 | Dr Kurt Pflughoeft Kurt has been with Market Probe since 1999 and oversees the corporate marketing science and data mining division. He leads an experienced team of senior statisticians at Market Probe. Kurt’s team ensures that the company can provide actionable information and recommend strategic initiatives for its global clients. Read the full biography here. |
Data Mining for Market Research Projects OR Why it's time to get the metal detector out By Dr Kurt Pflughoeft - 16th September, 2010
In the midst of this dour economy one product which has experienced increased sales is the metal detector.1 For many buyers, searching for buried treasure supports the dream of getting rich quick. Similarly in business, a technology that is often glamorized is data mining - the metal detector of corporate databases.
For many market research projects, especially trackers, a large amount of money is spent on data collection. Emphasis is placed on seeing data and simple reports in near real-time, supported by internet and wireless technologies. Proportionally little money or time is allocated to conduct advanced analysis. Consequently, the data that have been amassed from these surveys and other sources may contain untapped information.
Data mining may offer a solution for those who want to explore their data sources more fully. Workflows can be created within data mining packages to expedite advanced analytics and establish background processes to search for relationships.2 Users often dream of finding that one golden relationship; i.e., a great find which dramatically affects the organization in a positive manner.
 |
Just like metal detectors, the company is more likely to find “lesser-valued coins” with their data mining efforts; i.e., smaller incremental insights into the data. |
 |
But, just like metal detectors, the company is more likely to find “lesser-valued coins” with their data mining efforts; i.e., smaller incremental insights into the data. Although small gains may not seem as alluring, they should not necessarily be discounted. Many times it only takes a small gain for most organizations to reap a disproportionate competitive advantage. For example, information which leads to a ten percent increase in customer retention can be quite valuable to an organization.
Before embarking on a data mining project, the company’s data should be organized in some manner, preferably a corporate database or warehouse. These data repositories can be accessed directly by a data mining package or they can be used to extract a smaller subset of data known as a data mart. Data marts may contain only a random subset of the records and potentially only those fields deemed necessary for analysis via data mining.
Getting clean data is always a challenge even if the company has a corporate database. A normalized database can help preserve the integrity of the data, but the database itself can not guarantee the data quality.3 The quality of the data is only as good as its source and the processes which manipulate it. Consequently, many data mining efforts find that considerable work needs to be done in scrubbing the data so that it is suitable for analysis. Although analysts and data miners desire to have pristine data, do remember that many analysis methods can handle some degree of measurement error.
The definition of data mining varies from person to person. Some view data mining as the application of one or more statistical and non-statistical techniques. Others view data mining as a process that starts out with understanding the client’s business and ends with the deployment of a solution. However, by focusing on solely techniques or software, it would be hard to realize the full benefits of data mining.
The process of data mining contains several steps as defined by CRISP-DM framework. Readers interested in this holistic approach to data mining should see the CRISP-DM web site for further information.
To demonstrate the benefits of data mining, an illustrative case study will be used.
Management for a large company had created an annual customer feedback report using the data from several surveys. Part of this report compares annual attitudinal scores with the company’s total revenue, as shown in Figure 1. In the graph, the sad and possibly illogical pattern is that as revenue increased, customer attitude scores decreased. Needless to say, management was concerned and asked that the general link between customer attitudes and business outcomes be more thoroughly investigated. Management had many questions such as 1) Is the direction of the relationship correct? 2) Can a change in the customer satisfaction score be related to a change in actual business outcomes? 3) Is satisfaction the right attitudinal measure? Or should it be value, loyalty, etc..? 4) What statistic should be used to summarize customer attitudes? Averages? Top Box? Factor Scores?
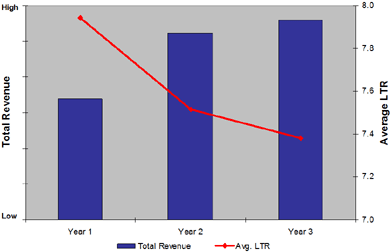
Figure 1 – Annual Customer Feedback
Additionally, the company was considering alternative formulations of revenue such as: average revenues, total revenues, revenues less credits, etc... These variations of business outcomes coupled with alternative attitudinal measures were being considered for many data partitions. The partitions consist of several customer segments, product lines and time frames. When pondering the number of possible combinations within the data partitions, it became clear that a tool was needed to expedite this search.
Within most data mining packages it is possible to set up graphical workflows for many of the operations. Figure 2 shows a high-level view of a generic workflow process but it should be pointed out that the order of some of these operations can be varied. Generally, the workflow iterates through the operations until a good model is found; in our case, a customer attitude measure which is best related to a business outcome.
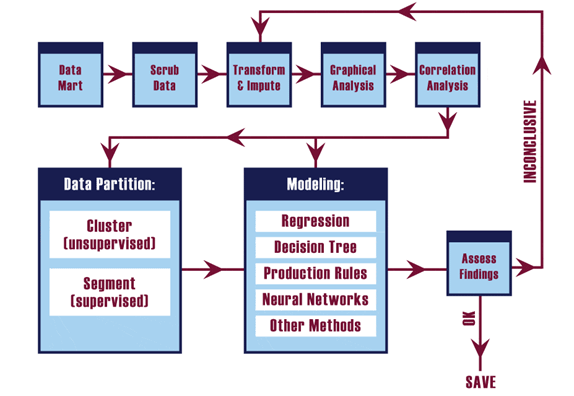
Figure 2 – Possible Data Mining Workflow
Obviously, some planning was involved in the design of the actual workflow for this case. It wasn’t just a matter of pushing a button without any objective or added insight/direction and then magically a relevant relationship appeared. However, the data mining package did allow the researchers to consider many more possibilities than could have been accomplished by traditional methods. This exploration also proved to be quite useful when answering management questions concerning the consideration of alternative formulations for the linkage of attitudes on business outcomes.
Initial results from the data mining effort showed that the reverse relationship in Figure 1 was due to Simpson’s Paradox.4 Simpson’s Paradox occurs when the partitioned data is blindly grouped together without formal analysis. Breaking down the year-to-year relationship into a monthly one by product lines revealed a direct positive relationship. The relationship between ‘Likelihood to Recommend’ and revenue for a particular line of business is shown in Figure 3.5
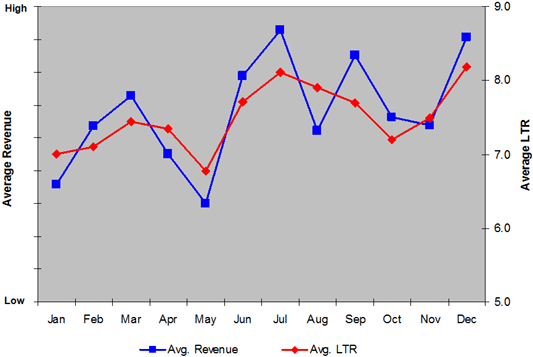
Figure 3 – Monthly Relationship between Customer Attitudes and Revenues
The benefits of establishing a relationship between attitudes and revenue were many. First, it was possible to estimate how much revenue may change due to changes in customer attitudes. Second, the relationship showed both management and front-line employees that customer service is important and does affect the bottom line. Finally, a closer examination of the monthly fluctuation in scores showed the need of implementing policies which are consistently followed.
So data mining may not find doubloons in the corporate database or lead to automated analysis, but this technology does offer tangible benefits to organizations. Incremental findings and improvements derived via data mining can lead to competitive advantages. As the organization gains more experience with data mining, they will learn other ways to leverage their data repositories.

1 In tough times, dreams of easy money. Disillusioned drive up sales of metal detectors, lottery tickets in downturn.
2 Workflows may be referred to as knowledgeflows in data mining.
3 See “Database normalization” in Wikipedia.
4 See “Simpson’s Paradox” in Wikipedia.
5 Be careful of spurious correlations such as the strong relationship between butter production in Bangladesh and the S&P 500 stock index.

Kurt Pflughoeft
Comments on this article

Want to share your thoughts...?
In this context, data mining is synonymous with exploratory analytics, and all statistical analytics rests on underlying models. It just in the nature of things. The question is: is there interesting potential data models that when applied will lead to new market or business insight? The answer is sometimes. However, it should be noted that the effective application of exploratory analytics is as much art as it is science and as much luck as it is systematic. Finding the key is always the problem.
NOTE: Please note that this board is moderated, and comments are published at the discretion of the site owner.
|