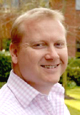 | Jeremy Griffiths Jeremy has been with Maritz Research since 1998 and is Director of Marketing Sciences for Europe. He is involved in projects across all sectors and methodologies and brings this broad expertise to provide analytical and statistical consultancy to recommend the most appropriate methodology for all our research projects. Read the full biography here. | Market Segmentation: Best practices and keys to success a beginners guide By Jeremy Griffiths - 1st April, 2010 Introduction The concept of market segmentation was formalized by Wendell Smith, who published a seminal article in The Journal of Marketing in 1956. He argued that in place of mass markets, goods would “find their markets of maximum potential as a result of recognition of differences in the requirements of market segments”. By the 1990s, questions arose about the effectiveness of market segmentation, and its future. With the advent of “one to one marketing,” segmentation seemed perhaps less useful. Segmentation continues to occupy a useful middle ground between mass marketing and one to one marketing. However, many poorly designed or ill-managed segmentation studies disappoint marketers and are frustrating to the clients who buy them and the consultants who supply them. The good news is that there are some concrete things you can do to keep control of segmentation studies and to maximize their chances of success. These fall into five categories: 
| | 1. | Study design | | 2. | Measurement | | 3. | Analysis | | 4. | Reporting | | 5. | Communications | 1. Study Design There are several criteria that the results of your segmentation should achieve. Your segments should be: | Identifiable | – distinctly different on easily measurable variables | | Substantial | – large enough to matter | | Accessible | – reachable through marketing, advertising, and distribution efforts | | Stable | – persist over time | | Responsive | – respond to efforts aimed at them | | Actionable | – differences are able to guide marketing decisions | | Creating a successful segmentation study, involves careful preparation and planning. To maximise the likelihood for success, your segmentation project should be a collaborative effort between client and research agency. Importantly you should collaborate internally with the many stakeholders who stand to benefit from the implementation of a successful segmentation strategy. For example you might want to engage with your marketing, operations, NPD, HR and IT functions etc. to consider the following: |  | What will the segmentation achieve for your business? | |  | What business outcome are you trying to influence? | |  | What decisions will you make with the information? | |  | Who will use the outputs of the segmentations? | |  | How often will the results be refreshed? | |  | Do you want to link the results to other internal data sources? | |  | Do you have in mind what the results might look like already? | |  | How many customer segments can you realistically work with? | By addressing these questions upfront you will be best placed to design the key structural components of the segmentation study. The main topics here are having an adequate sample size to profile the segments and selecting the best basis and profiling variables to accomplish the study objectives. Sample-size It is important to consider the sample size for your study to ensure you have enough robust data to define differences in the features and descriptions of customers in each segment groups. We recommend 200 – 300 respondents per customer group in the final segment solution. Selecting Basis Variables An important stage in the planning and design of the study is the selection of basis variables. The basis variables are the specific survey attributes from which the segments are mathematically derived, and their selection can impact the design, analysis and interpretation of a study. However, putting too many basis variables into the mix dilutes resultant segments. Therefore it is important to consider carefully the objectives of your segmentation study and hence how the selection of basis variables might help or hinder your results. Analytical methods are becoming available to help the marketer identify the most useful variables to determine segment solutions. Whilst these techniques are not a panacea for the lazy segmentation designer, they do help identify which basis variables contribute most to the clarity of the solution. Selecting Profiling Variables Profiling variables are those survey attributes that are used to help describe the distinctive features of the segment solutions. Once the segments are chosen, it is the information provided by the profiling variables that allows a full understanding of what makes the segments tick, such as how they can be appealed to with new products, or what types of messages they are most likely to respond to. Therefore, including a variety of relevant profiling variables is critical. 2. Measurement Using discriminating measures for the basis variables is extremely important if you are trying to build a needs based segmentation. In many studies attitude-based segments are derived from Likert “agree-disagree” rating scales. The between-respondent biases observed in this type of rating scale can create havoc for segmentation algorithms, creating results that are neither well differentiated nor actionable. This between-respondent bias can be particularly strong in multi-country segmentation projects. Therefore you should consider self-standardising or constrained measures of attribute importance for needs-based segmentation are preferred. For example; |  | Method of Paired Comparisons | |  | Maximum Difference, or “Best-Worst” Scaling | |  | Discrete Choice Experimental Designs | These methods require respondents to make choices between attributes they like/need and attributes that they do not like/need. Forcing trade-offs like this is a very successful way of highlighting the differences between respondents and therefore is particularly valuable for segmentation studies and can perform very well in multi-country studies. It may not always be feasible to collect self-standardising measures. In these cases careful construction of rating scale items can improve the problems found with less well designed rating scales. This increases the chance that the segmentation will be successful in producing crisp, well-defined and robust segments. Examples of best practice for rating scale needs-based items include: |  | Keeping the items category-specific rather than general | |  | Using items that force respondents to make tradeoffs (e.g. “I would rather have faster service than pay a slightly lower price”). Note that this is a rating scale equivalent to the method of paired comparisons. | Not all segmentation projects are solely based on customer attitudes, needs and wants. Some of the most successful segmentation solutions combine attitudinal and behavioural information about customers and under the guidance of an expert, it is possible to combine many different data types and create robust, reliable results. 3. Analysis Analysing segmentation results can be a time intensive, iterative effort best accomplished by members of both the research provider’s and the client’s staff. Ideally both researchers and marketers are involved, since the selection of the best solution must be shared between these two functions. Selecting the Right Segmentation Technique Because different segmentation basis variables (demographics, psychographics, needs, etc.) have different measurement properties, they require different mathematical models to create segments. Thus factor analysis followed by a convergent cluster analysis routine works well for attitudinal or psychographic data. Demographic data typically consists of categorical variables, making latent class analysis or PAM methods (Partitioning Around Medoids) appropriate for demographic segmentations. On occasions where the objective might be to find segments that differ with respect to one variable, but that are defined with observable, demographic variables, tree-based algorithms, like CART, CHAID or Randomforests can be used. Developing a List of Potential Solutions The mathematical methods used to divide respondents into segments do not produce DEFINITIVE statistical tests that show the number of segments there are, rather, the methods provide only statistical hints and evidence about which PATTERNS of segmentation that are not viable. Therefore it is important to run the analysis several ways to search for convergent validity. Segmentation techniques lack the “hard” validity measures of some multivariate techniques, but there are numerous statistical clues that can guide judgment. The point of this exercise is to avoid false discovery, or the acceptance of a solution that on its face appears valid, but is less statistically robust than alternatives. After running multiple iterations to assess statistical robustness of solutions, the researcher usually ends up with three to five segmentation plans that are statistically viable. The task is then to choose between these candidate solutions and select the one wining solution that meets the objectives of the study most effectively. Once shown the short set of viable segmentation solutions, clients use their insight and judgment to select the segmentation solution to use for final reporting and for subsequent marketing efforts Validating the Findings There are a variety of statistical methods used to maximize the validity of segmentation studies. Analytically, methods that offer convergent validity by using many iterations of the segmentation algorithms, each with slightly different starting conditions to produce robust solutions, are preferable. For post-study validation, follow up studies either quantitative or qualitative that test how segments fare when applied to a new sample of respondents can be useful. 4. Reporting Statistical evidence rarely points to a single segmentation solution, and there are often several statistically viable solutions to choose between. Whilst it is simple enough to produce a set of summary tables (or crosstabs) to review segment solutions, a better approach is to make use of technology and create a “Segmentation Viewer”. Segmentation Viewers can be easily set up in Excel to enable all team members, even the non-technicians, to interact directly different segmentation solutions and compare and contrast them in a user-friendly way. By creating an easy to use segmentation viewer, the process of selecting and describing the key features/winning solution from a range of candidate solutions is made all the more easy. 5. Communication More so with segmentation than other types of research, a consultative partnership is needed to guide a successful outcome. An important component of this approach is defining the workflow process up front, and scheduling specific meetings to provide updates at crucial times. As a starting point, confirm objectives and then describe the hypothetical outcome of the study. You could role play or enact the actions that you might take based on hypothetical output. This is useful way to map a process for developing action steps. By seeing how your organisation might work with hypothetical output, you can refine the segmentation study design or suggest ways to better leverage the segmentation investment by considering other initiatives. At the completion of the study, the actual results may show little resemblance to the hypothetical “practice” results but the client team has been through a dress rehearsal and is ready to apply an agreed upon process for taking action on the information. Summary Segmentation is not voodoo analytics. It is simply the mathematical process of dividing people into groups that are relevantly different, and then profiling the groups on the available descriptors to assess their validity. Segmentation studies should follow a process (see figure below) to ensure key decisions are made early and with the input of the right people. This process should be transparent, involving the client’s management in critical decisions, especially about the choice of segmentation bases, the goals for the segmentation, and the selection of a specific segmentation solution from among the set of statistically viable candidate solutions. Management buy-in is critical to the success of segmentation studies, because unless the intended users of a segmentation study understand and credit its results, it will be useless. 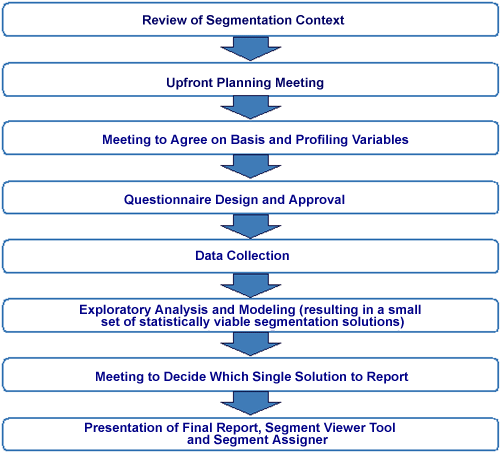 References Smith, Wendell. 1956. “Product Differentiation and Market Segmentation as Alternative Marketing Strategies”, Journal of Marketing, July, pp. 3-8 Frank, R.E., Massy, W.F. & Wind, Y. 1972. “Market segmentation”. Englewood Cliffs: Prentice-Hall.  Jeremy Griffiths
Comments on this article

Want to share your thoughts...?
NOTE: Please note that this board is moderated, and comments are published at the discretion of the site owner.
|